Nebraska Transportation Center
Scott Schrage, July 2, 2021
Model predicts lane changes, could inform driver-assist systems
It’s a high-speed, high-stakes hazard familiar to anyone who’s spent time driving on the interstate.
A car in the right lane lingers beside the opening between your vehicle and the SUV directly ahead of you in the left. The impromptu caravan is cruising along at the same speed, the illusion of stasis broken only by the blurring of the road and sleepy passage of the surrounding terrain.
Having driven this scene before, you keep an eye on the right-lane lingerer, ready for them to merge into the gap separating you from the SUV. But as the seconds pass, your vigilance wanes. As if on cue, the lingerer darts left with the suddenness of a slalom skier and forces you to brake, lest their recklessness become a wreck.
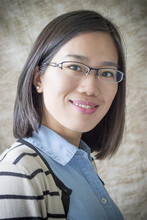
Zhao
Li Zhao and her colleagues at the Nebraska Transportation Center know the hazard, too. So the researchers have developed a new model that can read between the white lines to help predict when vehicles will change lanes. Their efforts could ultimately help give advanced driver-assistance systems — the vehicle-housed tech designed to anticipate threats and correct for human error — more lead time to react and, ideally, protect.
“If I know the intention, that the vehicle is going to abruptly cut in, I may have a corresponding reaction,” said Zhao, a postdoctoral researcher with the center. “I may slow down a little bit, or I may make another lane change to avoid a potential rear-end crash.”
The team built its model on data from roughly 3,000 vehicles outfitted with front-facing cameras and various sensors. In the early 2010s, the owners of those vehicles drove their regular routes for two months as part of a project funded by the U.S. Department of Transportation, which eventually made the naturalistic driving data available to the public.
To inform the model, Zhao began compiling data from every scenario in which one of those vehicles was following no more than 400 feet, or 3.5 seconds, behind another on the freeway. In some cases, both the front and trailing vehicle were in the same lane before one merged into an adjacent lane; in other cases, one vehicle merged from an adjacent lane, so that both ended up in the same.
Zhao tagged multiple variables that could act as telltales of a driver planning to change lanes: the distance between vehicles, their relative speed, their lateral positions, a subtle turn of the front vehicle’s nose. She then trained a model to analyze the values of those variables at every tenth of a second over a six-second span, from five seconds before a lane change until one second after.
At each of those 60 increments, the model compares the value of each variable — say, a 10-foot decrease in the distance between vehicles — against the estimated likelihood that the value will occur before a lane change. When all of those variables reach values that indicate the maximum likelihood of a lane change, the model flags the lane change as imminent.
Though it varies a bit across conditions, the model is able to predict a lane change about one second before the center of a vehicle crosses a dividing line on its way to another lane.
“One second ahead of time, we start to become confident that the driver is going to make a lane change,” Zhao said. “That may not mean much to a human driver, but we are talking about automated vehicles or advanced driver-assistance systems.
“So they can use the lead time to either improve their system, or they can design some extra safety precautions — alerts or warnings, like a crash warning system — to automatically slow down the vehicle or help the driver make some decisions.”
The team picked up on some other interesting trends, too. The average lane change, for instance, took between 0.55 and 0.86 seconds. Drivers actually tended to take less time merging into a lane behind a vehicle (the 0.55 seconds) than when changing lanes to get out from behind one (the 0.86). The faster a lane change, the more lead time a driver-assistance system needs in order to compensate, making the distinction a potentially useful one.
Zhao said having access to naturalistic driving data gives her greater confidence in the validity of the model, which she’s optimistic might also be applied to other, richer datasets from vehicles equipped with more sensors and cameras.
“I think the contribution of these results is to provide some background knowledge, technical data or support for the developers of these advanced driver-assistance systems and automated vehicle technologies,” she said. “That should help drivers in risky situations and improve driver safety when those extreme situations occur.”
Zhao developed the model with Laurence Rilett, director of the Nebraska Transportation Center and professor of civil and environmental engineering, along with Mm Shakiul Haque, doctoral student in transportation systems engineering. The researchers detailed the development and results of their model in the journal Transportation Research Record.